Introduction
Artificial Intelligence (AI) has moved from the realm of science fiction to being a business imperative, offering companies opportunities for innovation and efficiency like never before. Yet, the journey toward AI integration is far from straightforward. For business leaders, the challenges are not just about picking the right algorithms or data sets; they are about steering the organization through financial, ethical, and even cultural roadblocks.
This article aims to explore these pressing challenges from a business management perspective, offering actionable insights to help leaders successfully implement AI into their operations. We will provide solutions and case studies that serve as a roadmap for those venturing into the complex yet rewarding territory of AI adoption. If you're considering adding AI to your business toolkit, this article will equip you with the knowledge to navigate the multi-dimensional challenges ahead.
1. Technical Expertise
1.1 Introduction
As AI technologies continue to evolve and become more intricate, the depth of technical expertise required to harness their full potential also increases. Businesses venturing into the realm of AI often find themselves at the crossroads of innovation and complexity. The rapid advancements in AI mean that the tools and techniques that were relevant a few years ago might now be obsolete. This dynamic nature of AI technology can be daunting for businesses.
One of the primary challenges businesses face is the lack of customization in off-the-shelf AI solutions. While these solutions offer a generic set of features, they often fall short when it comes to addressing the unique challenges and requirements of individual businesses. Every business has its own set of data, customer base, and operational nuances. Implementing a one-size-fits-all AI solution can lead to inefficiencies and missed opportunities. For instance, an AI model trained on generic data might not perform optimally when exposed to a company's specific data set. This necessitates the customization of algorithms, data sets, and models to ensure that the AI solution aligns with the business's objectives and operational intricacies.
1.2 Solutions
Training Existing Employees
Investing in training programs can equip current employees with the necessary skills to understand and implement AI solutions. This not only enhances the skill set of the workforce but also ensures that the AI implementation aligns with the company's vision and goals.
Hiring Specialists
Bringing onboard AI specialists or data scientists can provide the technical prowess required for seamless AI integration. These experts can guide the customization process, ensuring that the AI models are tailored to the business's specific needs.
Consulting with AI Vendors
Collaborating with AI solution providers or vendors can offer valuable insights into the latest trends and best practices in the industry. These vendors often have a wealth of experience in customizing AI solutions for various industries and can provide guidance on the best approach for a particular business.
1.3 Case Study: Munich Re's Approach to Overcoming Technical Expertise Challenges1
Munich Re, an established German insurance company with 140 years of history, confronted multiple challenges when attempting to integrate AI into its operations. The company grappled with issues that ranged from securing adequate financial resources to obtaining the specialized technical know-how needed for successful AI implementation. Another challenge was to ensure that their technology infrastructure could robustly support the nuances of AI solutions. Additionally, the rapid and evolving nature of AI technologies made it clear to Munich Re that a generic, one-size-fits-all approach wouldn't be effective. The intricacies involved in AI technology required them to be both agile and adaptive to fit it into their particular business model and operational complexities.
To navigate the labyrinth of challenges, Munich Re took a systematic and multi-pronged approach. They initiated their journey by setting up dedicated teams specifically focused on the continuous testing and development of digital initiatives, including AI technologies. They also recognized that their existing workforce was among their most significant assets and invested in extensive training programs to enhance their technical skills. This focus on upskilling ensured that the AI technologies would align well with their specific business needs. Additionally, Munich Re extended their knowledge base by collaborating with external AI experts and vendors, giving them a comprehensive understanding of current industry best practices that could guide their own customization processes.
Munich Re's experience serves as a valuable lesson for other businesses facing similar challenges in integrating AI technologies, especially those that revolve around overcoming business challenges. The company successfully demonstrated that going through the technical expertise gap does not only involve external collaborations and financial investments but also involves a focus on internal talent development. By committing to continuous learning and internal upskilling, Munich Re was able to tailor AI solutions to their specific operational needs while staying ahead of technological advancements. Their systematic, agile, and adaptive approach thus stands as a model strategy for other businesses aiming to not just overcome challenges in AI integration but also to turn these challenges into avenues for innovation and growth.
2. Resistance to Change
2.1 Introduction
When it comes to integrating AI solutions into a business, technology is often not the most significant hurdle; it's the people. Human beings are complex variables in any equation of change, especially when that change involves a shift in the very fabric of how tasks are performed and decisions are made. Resistance to change is a well-documented psychological phenomenon, and it's no different when AI enters the workplace. Employees may feel threatened by the perceived complexity of AI or fear that automation will render their roles obsolete. This resistance can manifest in various ways, from passive non-compliance to active sabotage, making it a significant challenge for businesses aiming for a smooth transition to AI-enabled operations.
2.2 Solutions
The key to overcoming resistance to change lies in proactive change management strategies. One effective approach is staff training that goes beyond merely teaching how to use new AI tools. The training should also focus on the "why" behind the change, helping employees understand the benefits that AI can bring to their roles and the organization as a whole. This can alleviate fears and create a sense of ownership and engagement among staff.
Creating a culture that embraces change is another crucial element. This involves leadership demonstrating a commitment to innovation and continuous improvement. Open communication channels where employees can express their concerns and ask questions can go a long way in dispelling myths and uncertainties surrounding AI. Moreover, involving employees in the decision-making process can also foster a sense of inclusion and control, making them more receptive to new technologies.
In addition to these internal strategies, external expertise can also be invaluable. For instance, change management consultants specializing in AI integration can offer insights and frameworks for effectively managing the human aspect of this technological shift.
By combining these approaches, businesses can not only successfully integrate AI into their operations but also turn their most unpredictable variable—their people—into their greatest asset in this transformation.
2.3 Money Talks
While the challenges of human resistance to AI are significant, they are set against a backdrop of rapidly increasing corporate investment in this transformative technology, as illustrated in the following graph.
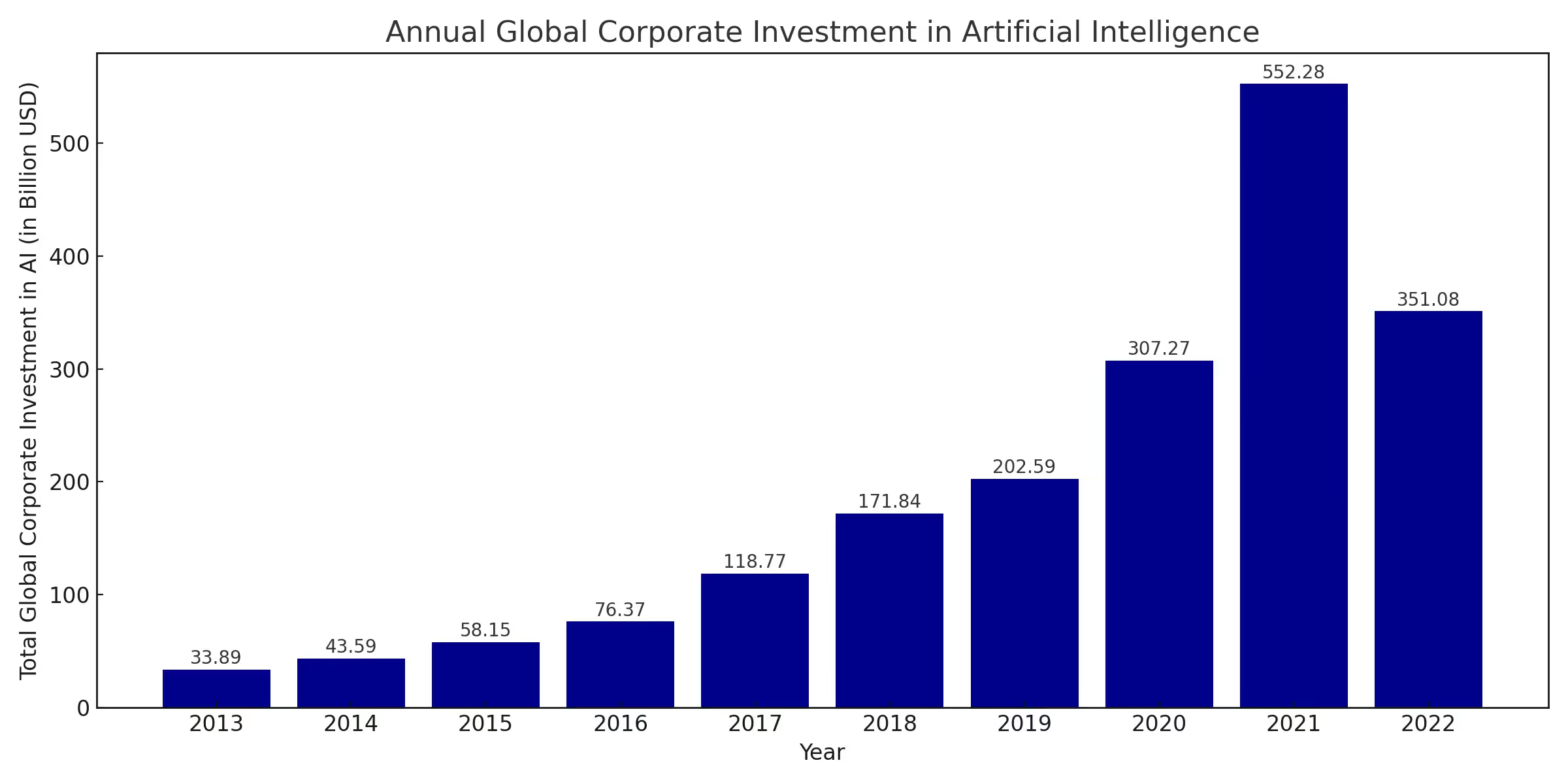
The surge in annual global corporate investment in AI, as depicted in the preceding graph, underscores the growing importance of this technology across industries. However, the sheer scale of financial investment is only part of the story. As significant as these investments are, they also bring to the fore the challenges of integrating AI into existing business operations—a transition that is not just technological but also deeply human. As outlined before, employees can be the most complex variables in this equation. The enormity of the investments shown in the graph highlights the urgency for companies to address not just the technical aspects of AI integration, but also the human factors that can make or break its successful adoption. Strategies for overcoming resistance—ranging from comprehensive staff training to creating an organizational culture that embraces change—are not just optional but essential. They complement the financial investments by ensuring that the technology is effectively utilized and that the human capital of the organization is aligned with this transformative shift.
3. Cost
3.1 Introduction
The cost of implementing AI solutions is a significant barrier, especially for small to medium enterprises (SMEs). While the allure of automating processes and gaining data-driven insights is tempting, the financial investment required can be daunting. The costs are not just limited to the initial setup but also include ongoing maintenance, data storage, and the hiring of specialized personnel. For SMEs that often operate on tight budgets, these costs can be a major deterrent.
3.2 Solutions
When it comes to funding AI projects, businesses have a variety of options. Government grants and subsidies are increasingly available for technological innovation, and these can significantly offset initial costs. Venture capital is another avenue, particularly for startups and high-growth companies, although this often involves relinquishing some level of ownership. Crowdfunding platforms offer another alternative, allowing businesses to raise small amounts of capital from a large number of contributors.
Strategic planning is crucial for maximizing the return on investment in AI. One approach is to start with a pilot program to gauge the technology's impact and potential return on investment. This allows businesses to make data-driven decisions about full-scale implementation. Scalability is another important consideration; opting for scalable solutions enables a company to start small and expand as results become evident, optimizing costs in the process. Open-source AI tools offer a cost-effective alternative but may require more specialized expertise to implement and maintain. Regular cost-benefit analyses can ensure that the investment in AI is justified, and for those who can't afford in-house expertise, outsourcing remains a viable but challenging option, given the risks associated with data security and quality control.
4. Data Availability and Quality
4.1 Introduction
Data is the lifeblood of any AI or machine learning algorithm. Without high-quality data, even the most sophisticated AI models are rendered ineffective. The importance of data in AI cannot be overstated; it's akin to fuel for a car. However, obtaining useful, high-quality data is often easier said than done. Many businesses find themselves grappling with the challenge of not just collecting data, but ensuring that it is relevant, clean, and ready for use.
4.2 Solutions
Ensuring data quality and consistency is crucial for AI systems. Merging data from different sources often results in inconsistencies and errors that require substantial time and effort to clean. Failure to address these issues can compromise the AI model's integrity.
Data privacy and security add another layer of complexity. The integration of diverse datasets increases the risk of exposing sensitive information, requiring strict data protection protocols. Techniques like data anonymization and encryption can help, but balancing data utility and privacy is challenging.
Additionally, combining data from multiple sources can inadvertently create new levels of confidentiality or personally identifiable information, known as "upclassing" or "deanonymization," which poses significant security risks. If internal data is lacking or insufficient for the AI application, businesses can also consider using third-party data services. These services often provide pre-cleaned, high-quality data that can be readily integrated into AI algorithms. However, it's essential to ensure that any third-party data complies with relevant legal and ethical standards.
The challenges of data availability and quality are not insurmountable but do require a focused and strategic approach. By adhering to best practices and leveraging available resources, businesses can significantly enhance the effectiveness of their AI integrations.
5. Ethical and Legal Considerations
5.1 Introduction
In the age of artificial intelligence, ethical and legal considerations have become increasingly complex terrains to navigate. While AI offers unprecedented capabilities for automating tasks, analyzing data, and solving complex problems, it also raises significant ethical and legal concerns. These concerns span from data privacy and security to algorithmic bias and accountability. For instance, AI algorithms can inadvertently perpetuate societal biases, leading to discriminatory outcomes in areas such as hiring, lending, and law enforcement. Moreover, the use of AI in data analytics often involves the collection and processing of vast amounts of personal data, raising questions about user consent and data protection. The legal landscape is equally intricate, with regulations like the General Data Protection Regulation (GDPR) in the European Union setting stringent rules for data handling but often lacking clear guidelines for AI-specific issues.
5.2 Solutions
Addressing these ethical and legal challenges requires a multi-faceted approach. One effective strategy is to conduct ethical audits of AI systems. These audits involve a comprehensive review of the AI's data sources, algorithms, and outputs to identify any ethical risks such as bias or potential infringements on privacy.
Moreover, it's crucial that ethical considerations are not just the responsibility of the tech team but are understood across the organization. Senior leaders, procurement officers, and even frontline staff should have a basic understanding of AI ethics to vet ethical risks effectively (source).
In the legal domain, compliance with existing laws and regulations is a given, but businesses should also actively engage with policymakers to shape future legislation. For example, the healthcare sector is grappling with how to implement AI ethically and legally, and active collaboration between tech companies and regulators can help map out the challenges and suggest directions for resolving them (source).
Additionally, businesses can adopt AI ethics frameworks that provide guidelines for responsible AI use. These frameworks often include principles like fairness, accountability, and transparency, serving as a roadmap for ethical AI development and deployment.
5.3 Case Study: Addressing Gender Bias in AI Search Engines by UNESCO2
Search engines are often the first point of contact for information gathering, but they are not immune to societal biases. A research led by UNESCO examined how search engines can perpetuate gender biases through their algorithms. Specifically, queries such as "greatest leaders of all time" were found to overwhelmingly feature male figures, and an image search for "school girl" produced sexualized images of women and girls. This not only raises questions about the accuracy of search results but also has broader implications. The perpetuation of such biases in the digital space impacts various sectors such as education, employment, and social interactions, further entrenching gender inequality.
To confront this issue, UNESCO adopted a comprehensive strategy as outlined in its Recommendation on the Ethics of Artificial Intelligence. This global standard-setting instrument encourages the minimization of gender bias in both algorithmic development and the large datasets used for machine learning. The recommendation further underscores the need to avoid the replication of stereotypical representations of women in digital platforms. By following these ethical guidelines, search engine companies have the potential to make significant progress in reducing gender bias, serving as a model for other AI applications to adopt ethical norms.
This case study is particularly relevant when discussing ethical and legal considerations for businesses integrating AI solutions. It highlights the importance of acknowledging that AI is not an impartial tool; it reflects the biases present in society and can exacerbate them if not carefully managed. Companies should be aware of the ethical ramifications of AI algorithms and take proactive steps to mitigate risks, particularly when it comes to reinforcing societal biases. The UNESCO guidelines offer valuable insights into developing more equitable AI systems, and businesses can learn from this case study that ethical considerations are not just a matter of compliance but also a key aspect in building robust and responsible AI systems. Following such ethical guidelines can contribute to risk mitigation and foster the development of more just and equitable digital platforms.
Final Thoughts
As we stand at the crossroads of technological revolution and human evolution, one truth is irrefutable: Artificial Intelligence isn't just a tool; it's a mirror reflecting our collective complexities—technical, ethical, and even existential. Businesses cannot afford to be passive bystanders in this transformative saga. Overcoming the challenges of technical expertise, resistance to change, financial burdens, data integrity, and ethical dilemmas isn't optional; it's a ticket to survival, a passport to relevance in an AI-driven future.
Each challenge echoes larger societal issues. Your algorithm may be devoid of human error, but can it be free of human bias? The AI that automates your mundane tasks today could be the tool that questions your ethical decisions tomorrow. So, as you unfurl your roadmaps and draft your strategic plans, consider this: integrating AI is not just about changing your technology; it's about questioning who you are as an organization, what you stand for, and how you'll evolve to meet the questions that we don't yet know how to ask. This isn't merely a technological shift; it's a philosophical awakening. Dare to engage.
