Introduction
In the ever-evolving realm of AI and machine learning, two pivotal technologies, Automated Machine Learning (AutoML) and XOps, are redefining the landscape with their remarkable impact on development efficiency and effectiveness. AutoML is revolutionizing the way AI and machine learning projects are undertaken by automating critical tasks such as exploratory data analysis, algorithm selection, training, and model optimization. This process, involving low-code or no-code platforms, streamlines the journey from data ingestion to the deployment of models, significantly enhancing the scalability and efficiency of AI projects.
On the other hand, XOps, encompassing practices like ModelOps, offers a structured approach to deploying models in a production environment. This set of practices addresses the challenges inherent in AI/ML projects, such as data inconsistency, labor constraints, and model complexity. By applying software engineering principles of Continuous Improvement and Continuous Delivery, XOps ensures consistency, reusability, and scalability, making it a cornerstone for successful AI implementations.
Together, AutoML and XOps are providing organizations with a powerful combination to extract more business value from AI projects rapidly and securely. They serve as the backbone for tools and processes that allow experimentation and deployment of AI models with remarkable efficiency, catering to the needs of a digitally transforming business world. As organizations continue to invest in AI, the integration of AutoML and XOps is becoming increasingly crucial, paving the way for smarter, more efficient AI solutions that drive intelligent, data-driven business decisions.
Understanding AutoML
Automated Machine Learning (AutoML) is revolutionizing AI by automating crucial machine learning tasks, enhancing efficiency, and unlocking new insights from data. It includes algorithms and tools that automate the training and tuning of models, allowing data scientists to focus on more creative tasks. AutoML improves data analysis accuracy by intelligently training models and exploring different feature engineering and hyperparameters. Its automation ensures models are properly trained and optimized, using efficient search algorithms for effective model searches. AutoML thus enhances the overall quality of results, uncovering trends or relationships in data and elevating the scalability and effectiveness of AI projects.
Exploring XOps
We’ve seen how AutoML plays a pivotal role in the evolving landscape of AI. In this context, XOps emerges as a transformative approach, building upon Development and Operations (DevOps). XOps, encompassing technologies like DevOps, DevSecOps, AIOps, MLOps, GitOps, and BizDevOps, aims to automate processes and shorten development cycles, enhancing software quality and delivery. Its key components, DataOps and MLOps, streamline data analytics and machine learning model deployment, aligning with business objectives. XOps promotes collaboration, automates operations, and ensures reliability, enhancing efficiency and customer experiences. Integrating XOps with AutoML technologies fosters operational excellence in AI projects, setting the stage for future innovations.

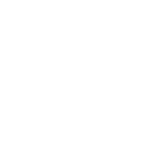
The Synergy of AutoML and XOps
The collaboration of AutoML and XOps significantly enhances AI development by streamlining both model creation and deployment. AutoML automates the model building process, efficiently handling tasks like data processing, feature selection, and algorithm optimization. This reduces the time and expertise required to develop accurate models. Concurrently, XOps practices, particularly ModelOps and MLOps, ensure these models are rapidly and reliably integrated into production environments. They address challenges like model maintenance, scaling, and compliance, facilitating continuous delivery and improvement. Together, this synergy reduces operational complexities and accelerates the deployment of robust, scalable AI solutions, which are crucial for success in a digitally transforming world.
Case Studies and Real-World Applications
In the realm of AutoML and XOps, several case studies demonstrate their profound impact on business activities:
1. Consensus Corporation: This company significantly expedited its fraud model deployment time from 3-4 weeks to just 8 hours, marking a 90% decrease. This rapid deployment allowed for quicker adaptation to emerging fraud techniques, illustrating AutoML's ability to enhance responsiveness and efficiency in critical business operations [1].
2. Capgemini Engineering: In the Hybrid Intelligence team, XOps was effectively utilized to integrate next-generation technologies like Digital Twin, Knowledge Graphs, EdgeML, and Quantum Computing. They created frameworks for these technologies, improving delivery standardization, turnaround speed, and solution quality. This approach enabled the adoption of emerging technologies, accelerating innovation. The project highlighted the importance of evolving XOps practices to encompass future technologies, showcasing XOps as a crucial factor in the successful industrialization of advanced technological solutions [2].
These examples reveal AutoML and XOps' transformative potential, showcasing their capacity to accelerate critical processes, improve accuracy in predictions, and democratize AI capabilities across diverse business sectors.
Challenges and Considerations
In the integration of Automated Machine Learning (AutoML) with XOps, organizations face a complex landscape of challenges. Despite AutoML's potential to democratize data science, its application in real-world settings is often hindered by limitations such as its lack of customizability and transparency. Users find AutoML platforms overly rigid, restricting their ability to fine-tune or adjust automated processes. Moreover, the inherent "black-box" nature of AutoML adds another layer of complexity, making it difficult to evaluate outcomes and understand underlying processes. However, practitioners are finding ways to adapt, setting clear goals for AutoML's capabilities and developing workarounds to maximize its practical usability, such as manually validating outcomes and assessing the dynamics of AutoML’s processes. These challenges underscore the need for ongoing improvement and innovation in the field for successful AutoML and XOps integration.
Future Implications
The integration of AutoML and XOps is poised to significantly influence the future trajectory of AI development. Market analysts forecast rapid growth in both AutoML tools and MLOps in the coming years. AutoML is increasingly adopted by businesses struggling to hire data science talent or seeking to boost the efficiency of existing teams. These tools address critical tasks traditionally requiring data scientists' expertise, such as algorithm selection, model tuning, and data preparation.
Furthermore, the machine learning platform market is expected to undergo significant consolidation, with an uptick in acquisitions, mergers, and IPOs. This trend suggests a maturing market that is integrating and standardizing AI and ML tools for broader accessibility and application. Alongside this, the expanding scope of ML platforms will likely see enhanced MLOps capabilities, further integrating machine learning models into the development lifecycle managed by DevOps teams [3].
This evolution suggests a future where AI development becomes more streamlined, accessible, and efficient. The fusion of AutoML and XOps is likely to lower barriers to entry in AI, democratizing access to advanced technologies and fostering innovation across diverse industries. As a result, organizations can anticipate more robust, scalable, and user-friendly AI solutions, driving intelligent, data-driven decision-making and opening new horizons in technological advancement.
Final Thoughts
As we've explored in the article, the synergy of AutoML and XOps is a game-changer in AI development. This fusion is not just about enhancing efficiency; it's a transformative shift towards democratizing AI and making advanced technologies accessible across various industries. While challenges persist, particularly in integrating AutoML's automated capabilities with XOps' operational excellence, the future is promising. The anticipated growth in AutoML tools and MLOps, coupled with market consolidation, heralds an era of more robust, scalable, and user-friendly AI solutions. This evolution is set to drive intelligent, data-driven decision-making, unlocking new frontiers in technology and business innovation.